Data Labeling is the backbone of successful AI and machine learning models, where annotated data provides valuable insights. Choosing the right approach between human and automated data labeling can significantly impact the effectiveness of your models.
Data Labeling and data labeling tool play pivotal roles in driving AI advancements forward, making them essential components of any ML project.
In this article, we’ll dive into the pros and cons of each method, helping you make an informed decision for your data labeling needs.

1. Human Data Labeling: Precision with Context
Pros:
- Humans offer contextual understanding, ensuring accurate annotations even in complex scenarios.
- Expert human labelers can handle unforeseen cases, adapting quickly to new data patterns.
- Ethical considerations are upheld, reducing potential biases in the data labeling process.
Cons:
- Manual data labeling can be time-consuming, leading to longer project timelines.
- Subjectivity among human labelers may introduce slight variations, affecting model consistency.
- Scaling human labeling can be challenging and costly for large datasets.
2. Automated Data Labeling: Speed and Consistency
Pros:
- Automated data labeling significantly speeds up the process, making it ideal for large datasets.
- Consistency is ensured as the algorithms apply labels uniformly across the data.
- Automation provides cost-effectiveness and scalability for data-intensive projects.
Cons:
- Automated systems may lack contextual understanding and struggle with complex data.
- Dependency on initial training data quality can impact the accuracy of the labels.
- Ambiguous data points may pose challenges for automated labeling.
Making the Right Choice: A Hybrid Approach
Both human and automated data labeling have their strengths and weaknesses. In many cases, a hybrid approach is the key to achieving the best results. Leveraging the precision of human labelers alongside the efficiency of automated tools can strike a perfect balance. Start by automating the labeling process to handle large volumes of data quickly. Then, utilize human expertise to review and refine the automated labels, adding context and ensuring accuracy where needed.
Conclusion
Data labeling plays a vital role in the success of AI and machine learning models. Human labeling offers precision and adaptability, while automation excels in speed and scalability. By combining the two approaches, data scientists can harness the benefits of both to create accurate and efficient models. Evaluate your project requirements and choose the best-suited method for your data labeling needs, bearing in mind that a hybrid approach often yields the most promising outcomes.
In the end, the success of your AI endeavors largely depends on the quality of the labeled data, so choose wisely and embark on your data labeling journey with confidence.
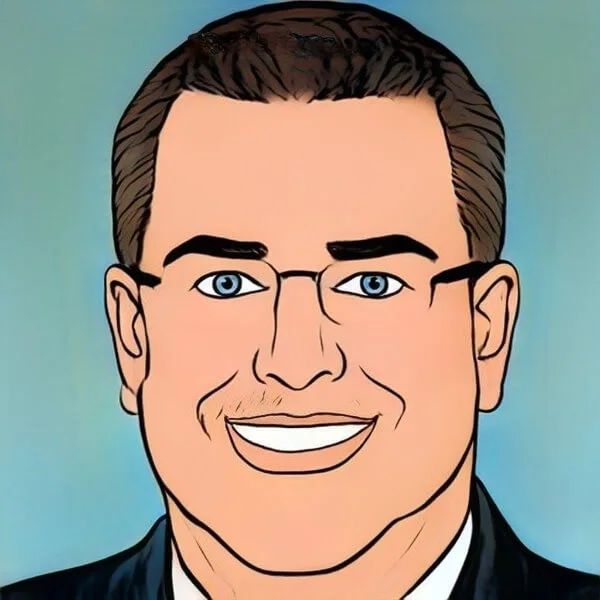
Julian is an expert in all things technology. He enjoys writing blogs about how to use the latest software and hardware on the market, while also providing advice for using existing technology more effectively. His favorite pastimes include reading comic books and playing video games.